How do researchers efficiently draw conclusions from large populations without examining every individual? The answer often lies in the powerful technique known as the "multi-stage sample." This method isn't just a buzzword; it's a cornerstone of effective research methodologies, allowing researchers to navigate complex populations with precision and efficiency. By breaking down the sampling process into manageable stages, multi-stage sampling minimizes logistical challenges and enhances the accuracy of research findings.
As we dive deeper into the world of multi-stage sampling, it's crucial to appreciate its significance in both academic research and practical applications across various fields. From sociology to health sciences, multi-stage sampling plays a pivotal role in generating insights that inform policy, drive innovations, and foster a deeper understanding of societal trends. In this comprehensive guide, we'll explore the mechanics of multi-stage sampling, its advantages, limitations, and real-world applications, providing you with a thorough grasp of this indispensable research tool.
Whether you're a seasoned researcher looking to refine your sampling techniques or a curious reader eager to understand how large-scale studies are conducted, this article will cater to your needs. We aim to demystify the concept of multi-stage sampling, presenting it in an accessible yet detailed manner. Join us as we embark on this informative journey through the intricacies of multi-stage sampling, ensuring you come away with a newfound appreciation for its role in the research landscape.
Table of Contents
- Introduction to Sampling
- What is Multi-Stage Sampling?
- Importance of Multi-Stage Sampling
- Steps in Multi-Stage Sampling
- Advantages of Multi-Stage Sampling
- Limitations and Challenges
- Applications of Multi-Stage Sampling
- Multi-Stage Sampling vs. Other Methods
- Case Studies
- Real-World Examples
- How to Conduct a Multi-Stage Sample
- Ethical Considerations
- Frequently Asked Questions
- Conclusion
Introduction to Sampling
Sampling is a fundamental aspect of research methodology, essential for drawing meaningful conclusions about a larger population without the need to study every individual. It involves selecting a subset of individuals from a population to estimate characteristics of the whole group. Sampling techniques can be broadly classified into probability and non-probability sampling, each with its unique advantages and applications.
Probability sampling ensures that every member of the population has a known, non-zero chance of being selected, which helps produce representative samples and allows for the generalization of results. Common probability sampling methods include simple random sampling, stratified sampling, cluster sampling, and systematic sampling. On the other hand, non-probability sampling does not guarantee that every individual has an equal chance of being selected, often relying on subjective judgment. This category includes techniques such as convenience sampling, judgmental sampling, snowball sampling, and quota sampling.
As researchers navigate the challenges of studying large and diverse populations, sampling becomes an invaluable tool. It reduces the time, cost, and effort required to collect data while maintaining the integrity and accuracy of the research. Sampling allows researchers to focus their resources on a manageable subset of the population, providing a practical solution to the logistical constraints of large-scale studies.
What is Multi-Stage Sampling?
Multi-stage sampling is an advanced probability sampling technique that divides the sampling process into multiple stages, allowing researchers to systematically reduce the size of the sample until they reach the desired level of granularity. This method is particularly useful when dealing with large, geographically dispersed populations, where other sampling techniques may be impractical or inefficient.
In a multi-stage sample, researchers begin by selecting a sample of primary units, which are often larger clusters such as districts, schools, or households. Within each primary unit, secondary units are chosen, followed by tertiary units, and so on, until the final sample is obtained. This hierarchical approach helps researchers manage the complexities of large populations while maintaining the statistical rigor of probability sampling.
The multi-stage sample is versatile and adaptable, allowing researchers to tailor the sampling process to the specific needs of their study. By using a combination of different sampling methods at each stage, such as stratified sampling or cluster sampling, researchers can enhance the representativeness and accuracy of their results. This flexibility makes multi-stage sampling a popular choice for large-scale surveys, social research, and public health studies.
Importance of Multi-Stage Sampling
The importance of multi-stage sampling cannot be overstated, as it addresses several challenges associated with traditional sampling methods. One of the primary advantages of multi-stage sampling is its ability to efficiently manage large and complex populations. By breaking down the sampling process into smaller, manageable stages, researchers can save time, resources, and effort while still obtaining reliable and accurate results.
Furthermore, multi-stage sampling enhances the representativeness of the sample, reducing the risk of sampling bias. By using a hierarchical approach, researchers can ensure that every subgroup within the population is adequately represented, leading to more accurate and generalizable conclusions. This is particularly important in studies involving diverse populations with varying characteristics and needs.
Multi-stage sampling also offers increased flexibility, allowing researchers to adapt their sampling strategy to the specific requirements of their study. By incorporating different sampling techniques at each stage, researchers can optimize the balance between precision and efficiency, ultimately improving the quality of their research findings. The adaptability of multi-stage sampling makes it a valuable tool for a wide range of research applications, from national surveys to local community studies.
Steps in Multi-Stage Sampling
Conducting a multi-stage sample involves a series of carefully planned steps, each designed to ensure the representativeness and accuracy of the final sample. The process begins with defining the target population and determining the overall sample size required to achieve the desired level of precision and confidence in the results.
The first stage of multi-stage sampling involves selecting primary units, which are typically large clusters that encompass the entire population. These primary units can be geographical areas, such as regions or districts, or organizational units, such as schools or companies. The selection of primary units is usually based on a probability sampling method, such as simple random sampling or systematic sampling, to ensure that every unit has an equal chance of being selected.
In the second stage, researchers select secondary units within each primary unit, further narrowing down the sample. This stage often involves a different sampling method, such as stratified sampling or cluster sampling, to enhance the representativeness of the sample. The process continues with additional stages as needed, each time selecting smaller units within the previously chosen units, until the final sample is obtained.
Throughout the multi-stage sampling process, researchers must carefully monitor and adjust their sampling strategy to ensure that the final sample is both representative and manageable. This may involve revisiting earlier stages, refining the selection criteria, or adjusting the sample size to account for unforeseen challenges or changes in the study's objectives.
Advantages of Multi-Stage Sampling
Multi-stage sampling offers several advantages that make it an attractive choice for researchers dealing with large and complex populations. One of its primary benefits is the ability to efficiently manage the logistical challenges of large-scale studies. By breaking down the sampling process into smaller stages, researchers can significantly reduce the time, cost, and effort required to collect data, while still maintaining the accuracy and reliability of the results.
Another advantage of multi-stage sampling is its enhanced flexibility and adaptability. By incorporating different sampling techniques at each stage, researchers can tailor the sampling process to the specific needs of their study, optimizing the balance between precision and efficiency. This flexibility makes multi-stage sampling suitable for a wide range of research applications, from national surveys to local community studies.
Furthermore, multi-stage sampling helps reduce sampling bias by ensuring that every subgroup within the population is adequately represented. By using a hierarchical approach, researchers can ensure that the final sample accurately reflects the diversity and characteristics of the entire population, leading to more reliable and generalizable conclusions.
Limitations and Challenges
Despite its many advantages, multi-stage sampling is not without its limitations and challenges. One of the primary challenges researchers face is the complexity of the sampling process, which requires careful planning and coordination to ensure that each stage is executed correctly. This complexity can lead to potential errors and biases if not managed properly, making it crucial for researchers to have a thorough understanding of the sampling techniques and methods used at each stage.
Another limitation of multi-stage sampling is the potential for increased sampling error, particularly in studies with a large number of stages. As the sampling process progresses through multiple stages, the risk of accumulating errors increases, which can impact the accuracy and reliability of the final results. Researchers must carefully monitor and adjust their sampling strategy to minimize these errors and ensure the representativeness of the sample.
Additionally, multi-stage sampling can be resource-intensive, particularly in terms of time and cost. While the method is designed to manage large populations efficiently, the complexity of the sampling process and the need for multiple stages can still require significant resources. Researchers must balance the benefits of multi-stage sampling with the available resources and constraints of their study to determine the most appropriate sampling strategy.
Applications of Multi-Stage Sampling
Multi-stage sampling is widely used across various fields and research applications, owing to its versatility and adaptability. In social research, multi-stage sampling is often employed in large-scale surveys and studies, where researchers need to gather data from diverse populations spread across vast geographical areas. By breaking down the sampling process into manageable stages, researchers can efficiently collect data while ensuring the representativeness of the sample.
In the field of public health, multi-stage sampling is a valuable tool for conducting epidemiological studies and health surveys. Researchers can use multi-stage sampling to gather data on health behaviors, disease prevalence, and risk factors, allowing them to identify trends and patterns within the population. The method's ability to accurately represent diverse populations is particularly important in public health research, where findings can inform policy decisions and interventions.
Educational research also benefits from multi-stage sampling, particularly in studies involving schools, students, and teachers. By selecting clusters of schools and then sampling students or teachers within those clusters, researchers can obtain a representative sample that accurately reflects the educational landscape. This approach allows for the collection of data on student performance, teaching methods, and educational outcomes, contributing to the development of evidence-based educational policies and practices.
Multi-Stage Sampling vs. Other Methods
When comparing multi-stage sampling to other sampling methods, it's important to consider the specific needs and objectives of the study. Each sampling method has its own strengths and weaknesses, and the choice of method depends on factors such as the size and complexity of the population, the available resources, and the desired level of precision and accuracy.
Simple random sampling is one of the most straightforward and widely used sampling methods, where each individual in the population has an equal chance of being selected. While this method is ideal for smaller, homogeneous populations, it becomes impractical for large or geographically dispersed populations, making multi-stage sampling a more suitable choice.
Stratified sampling involves dividing the population into subgroups, or strata, based on specific characteristics, and then randomly sampling individuals from each stratum. This method ensures the representativeness of the sample and reduces sampling bias, but it can be resource-intensive and challenging to implement for large populations. Multi-stage sampling can incorporate stratified sampling at one or more stages, providing a more efficient and flexible alternative.
Cluster sampling involves dividing the population into clusters, such as geographical areas or organizational units, and then randomly selecting entire clusters for sampling. While this method is efficient for large populations, it can result in increased sampling error if the clusters are not representative of the entire population. Multi-stage sampling addresses this issue by allowing researchers to select smaller units within each cluster, enhancing the accuracy and reliability of the results.
Case Studies
To illustrate the effectiveness of multi-stage sampling, let's examine a few case studies that highlight its application in real-world research scenarios. These case studies demonstrate how multi-stage sampling can be used to gather accurate and reliable data from diverse populations, providing valuable insights for decision-making and policy development.
In one case study, researchers conducted a national health survey to assess the prevalence of chronic diseases and risk factors among adults. The study used a multi-stage sampling approach, first selecting a sample of regions, then selecting districts within each region, and finally sampling households within each district. This hierarchical approach allowed researchers to efficiently gather data from a representative sample of the population, ensuring the accuracy and reliability of the findings.
Another case study involved a large-scale educational assessment, where researchers aimed to evaluate student performance and learning outcomes across different schools. The multi-stage sampling process began by selecting a sample of schools, followed by sampling students within each selected school. This approach allowed researchers to gather data from a diverse and representative sample, providing valuable insights into the factors influencing student performance and educational outcomes.
In a third case study, researchers conducted a social survey to examine the impact of socio-economic factors on community well-being. The study used multi-stage sampling to select a sample of communities, followed by sampling households and individuals within each community. This approach ensured that the final sample accurately represented the diverse socio-economic characteristics of the population, allowing researchers to draw meaningful conclusions about the relationship between socio-economic factors and community well-being.
Real-World Examples
Multi-stage sampling is widely used in various real-world applications, providing valuable data for decision-making and policy development. One notable example is the National Health and Nutrition Examination Survey (NHANES) conducted by the Centers for Disease Control and Prevention (CDC). NHANES uses a multi-stage sampling design to collect health and nutritional data from a representative sample of the U.S. population, informing public health policies and interventions.
Another example is the Programme for International Student Assessment (PISA), an international survey conducted by the Organisation for Economic Co-operation and Development (OECD) to evaluate education systems worldwide. PISA uses multi-stage sampling to select representative samples of students from participating countries, providing valuable insights into student performance and educational outcomes across different cultures and contexts.
In market research, multi-stage sampling is often used to gather data on consumer preferences and behaviors. By selecting a sample of geographical areas, followed by sampling households and individuals within those areas, researchers can obtain a representative sample that accurately reflects the target market. This data can be used to inform marketing strategies, product development, and business decisions.
How to Conduct a Multi-Stage Sample
Conducting a multi-stage sample requires careful planning and execution to ensure the accuracy and reliability of the final results. The process begins with defining the target population and determining the overall sample size required to achieve the desired level of precision and confidence in the results.
The first stage involves selecting primary units, which are typically large clusters that encompass the entire population. These primary units can be geographical areas, such as regions or districts, or organizational units, such as schools or companies. The selection of primary units is usually based on a probability sampling method, such as simple random sampling or systematic sampling, to ensure that every unit has an equal chance of being selected.
In the second stage, researchers select secondary units within each primary unit, further narrowing down the sample. This stage often involves a different sampling method, such as stratified sampling or cluster sampling, to enhance the representativeness of the sample. The process continues with additional stages as needed, each time selecting smaller units within the previously chosen units, until the final sample is obtained.
Throughout the multi-stage sampling process, researchers must carefully monitor and adjust their sampling strategy to ensure that the final sample is both representative and manageable. This may involve revisiting earlier stages, refining the selection criteria, or adjusting the sample size to account for unforeseen challenges or changes in the study's objectives.
Ethical Considerations
When conducting a multi-stage sample, researchers must adhere to ethical principles to ensure the integrity and credibility of the study. Ethical considerations include obtaining informed consent from participants, ensuring the confidentiality and privacy of the data collected, and minimizing any potential harm to participants.
Informed consent is a fundamental ethical requirement, ensuring that participants are fully aware of the study's objectives, procedures, and potential risks before agreeing to participate. Researchers must provide clear and comprehensive information about the study and obtain voluntary consent from participants, respecting their autonomy and right to withdraw from the study at any time.
Confidentiality and privacy are also critical ethical considerations, as researchers must protect the identity and personal information of participants. This involves implementing measures such as data anonymization, secure data storage, and restricted access to the data, ensuring that participants' information is not disclosed or misused.
Finally, researchers must strive to minimize any potential harm to participants, ensuring that the study does not cause physical, psychological, or emotional distress. This involves carefully assessing the risks and benefits of the study, implementing measures to mitigate any potential harm, and providing appropriate support and resources to participants if needed.
Frequently Asked Questions
1. What is the main advantage of multi-stage sampling?
The main advantage of multi-stage sampling is its ability to efficiently manage large and complex populations by breaking down the sampling process into smaller, manageable stages. This approach reduces the time, cost, and effort required to collect data while maintaining the accuracy and reliability of the results.
2. How does multi-stage sampling reduce sampling bias?
Multi-stage sampling reduces sampling bias by ensuring that every subgroup within the population is adequately represented. By using a hierarchical approach and incorporating different sampling techniques at each stage, researchers can ensure that the final sample accurately reflects the diversity and characteristics of the entire population.
3. What are some common applications of multi-stage sampling?
Common applications of multi-stage sampling include large-scale surveys and studies in social research, public health, and education. The method is also used in market research to gather data on consumer preferences and behaviors, as well as in international assessments such as PISA.
4. What are some limitations of multi-stage sampling?
Limitations of multi-stage sampling include the complexity of the sampling process, potential for increased sampling error, and resource-intensive nature of the method. Researchers must carefully plan and execute each stage to minimize errors and ensure the accuracy and reliability of the results.
5. How does multi-stage sampling differ from other sampling methods?
Multi-stage sampling differs from other sampling methods in its hierarchical approach, which involves selecting samples at multiple stages. This method is particularly useful for large and complex populations, where other sampling techniques may be impractical or inefficient.
6. What ethical considerations should researchers keep in mind when conducting a multi-stage sample?
Researchers must adhere to ethical principles such as obtaining informed consent, ensuring confidentiality and privacy, and minimizing potential harm to participants. These considerations help maintain the integrity and credibility of the study and protect the rights and well-being of participants.
Conclusion
Multi-stage sampling is a versatile and powerful research tool that enables researchers to efficiently manage large and complex populations while maintaining the accuracy and reliability of the results. By breaking down the sampling process into smaller, manageable stages, multi-stage sampling minimizes logistical challenges and enhances the representativeness of the sample, ensuring that every subgroup within the population is adequately represented.
While multi-stage sampling offers numerous advantages, it also presents challenges that researchers must carefully navigate to ensure the success of their study. By understanding the intricacies of the method and adhering to ethical principles, researchers can harness the full potential of multi-stage sampling to gather valuable data and insights that inform decision-making and policy development.
As we continue to explore the world of research, multi-stage sampling remains an indispensable tool for understanding the complexities of diverse populations and uncovering the insights that drive progress and innovation. By embracing the power of multi-stage sampling, researchers can contribute to a deeper understanding of the world around us, ultimately making a positive impact on society and the future.
You Might Also Like
Understanding Rabies In Raccoons: Risks, Symptoms, And PreventionProtecting Yourself From Instagram Spam Messages: A Comprehensive Guide
Discovering The Art Of Tattoo Designs: Honoring Your Wife's Name
The Essential Guide To Understanding True Pacifist Requirements: A Path To Peaceful Living
Popping In Neck When Turning Head: Understanding Causes, Treatments, And Prevention
Article Recommendations
- 5e Class
- Is Blocking Considered Cheesing In Fromsoft Games
- French Mythological Creatures
- Echinacea For Sore Throat
- Forest Sunlight
- Winter Essentials Woman
- John Bennett Perry
- Monopoly Cars
- Opal Engagement Ring
- Midwife And The Baker

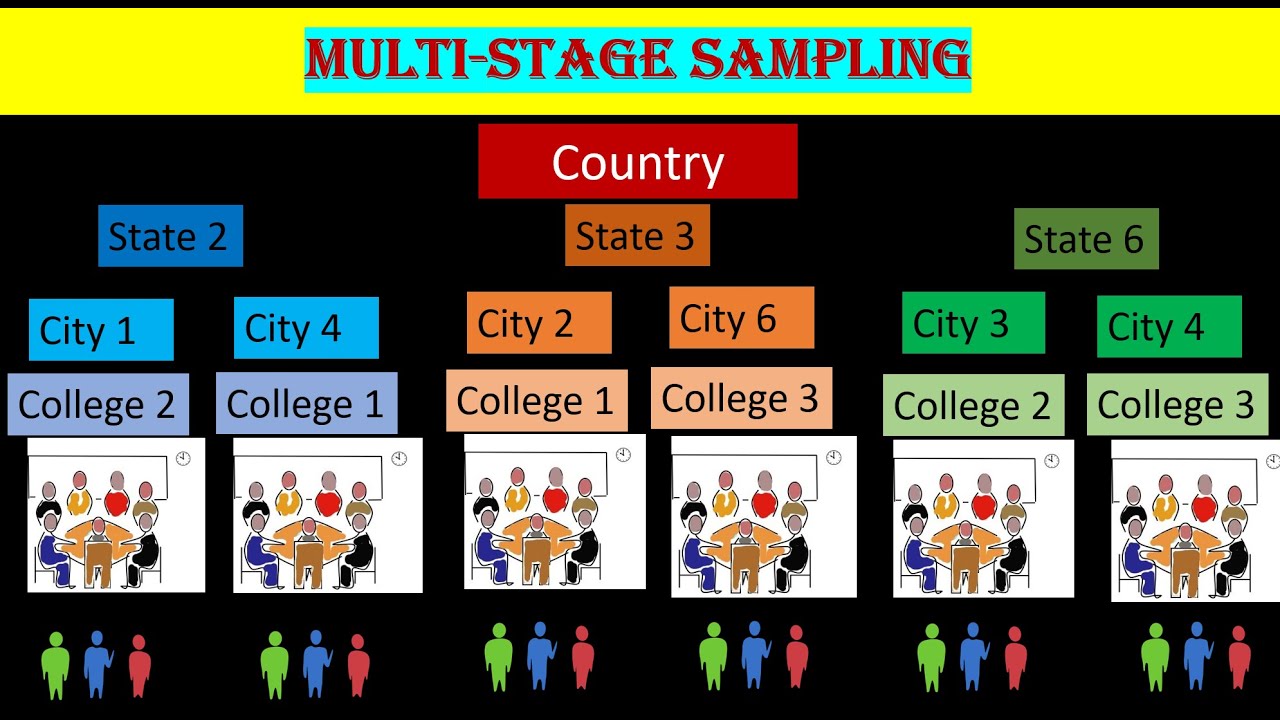